How much data should you and your team collect before making a decision? How do you determine the right data to collect?
I used to be in the camp of “only collect data that can impact your decision”. Would you change your decision based on data “X”? If not, then I would have argued that you don’t waste time and resources collecting data “X”. However, recently, my views have changed; today I believe that the answer is more nuanced.
The change in my perspective is due to advances in technology that have enabled us to analyze and utilize large data sets. Think of the increased utility of real-world data in drug development. Think of the utility of genetic data collected by testing kits now being sold to pharmaceutical giants. The truth is, there can be a utility to data that one may not foresee at the time of data collection. This trend will only become more prominent in the future as technologies such as machine learning continue to mature.
So, going back to my initial question, how do you and your team determine the right data to collect? I would propose you give it some thought before you make your choice.
Below is a simple framework that can help you with the thinking process:
First, define your KEY question(s). Clearly articulating the key question(s) and driver(s) for your experiment will provide the context for the next step.
After getting clarity on your objective, you can categorize the data you can collect from a given experiment into buckets, such as outlined below:
- Must-have: This is data that directly addresses your key question and is needed to move the project to the next decision gate.
- Nice-to-have: This is information that can provide additional support for your strategy, e.g. confirm a mechanism of action. If you don’t get this data, you can proceed with your project. However, this data would significantly enhance your position.
- Exploratory: This is information that you can collect to improve your understanding or capabilities for the future but does not directly relate to your key question, e.g. test a new (cheaper, faster) diagnostic to compare to the current gold standard.
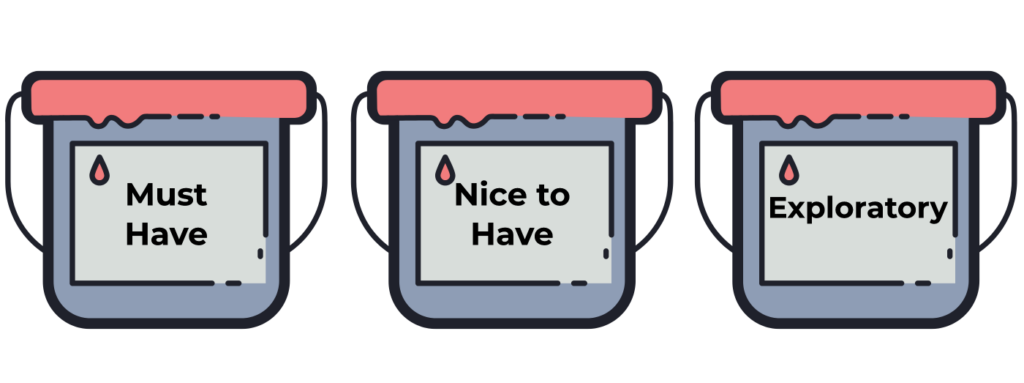
Based on the level of resources (people, money, time) available, you can determine what data you can practically collect. Make sure you document the rationale for the various data being collected!
Now keep in mind that you will never have a full or exhaustive data set. The goal of using the proposed prioritization scheme (or tiered approach) is to ensure that you collect must-have information to achieve your project milestones without being too short-sighted.
How do you tackle the question of data collection on your projects? I’d love to hear your perspective and experience.